
We propose a new kernel optimized to capture the logarithmic dependence of the effect on compound dose in biochemical systems. Different kernels can be used to express different structures observed in the data. A Gaussian process is completely defined by its mean and kernel functions.
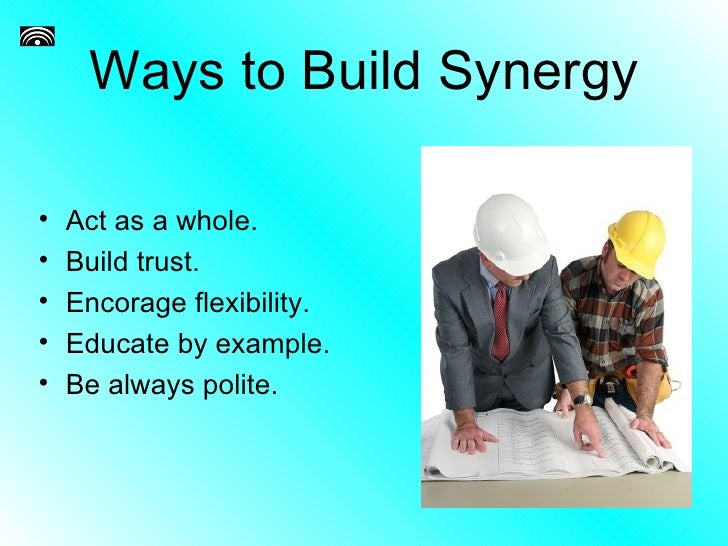
Our proposed model is based on Gaussian processes and is non-parametric. In particular, the kernel hyperparameters can change the prior distribution over functions. We follow common machine learning terminology where the parameters of the GP are called hyperparameters because they can be interpreted as such in a Bayesian setting. For comparison, our Hand-GP model has only 4 hyperparameters, one of which captures the noise level, i.e. Perpetrators cause a change of the half-maximal effective concentration, \(EC_\)).

In particular, they define perpetrator and victim drugs. Ratio-dependent synergy/antagonism happens when, say, antagonism is observed when the mixture is dominated by drug 1 and synergy when the mixture is dominated by drug 2. For example, antagonism can be observed at low doses at synergy and high doses. Level-dependent synergy/antagonism occurs when the difference (between non-interactive response and observed response) at low doses deviates from the difference at high doses. developed models for testing level-dependent and ratio-dependent synergy/antagonism. Loewe additivity and Bliss independence models often serve as bases for various extensions that incorporate more complex interaction patterns. present detailed theoretical comparisons of the popular null models including comparisons between the isoboles of the corresponding null models. However, there are other candidates such as the Highest Single Agent (HSA), the Tallarida and the recently rediscovered Hand model. The most common candidates for the non-interactive response models are the Loewe additivity and Bliss independence models. īy comparing the expected non-interactive (null) and observed responses, one can assess whether there is synergy or antagonism between two drugs. In ecotoxicology, this led to an understanding of how toxins interact and, in particular, how they can affect a human body. Understanding of synergy allowed the development of combination therapies which proved useful in various areas, including treatment of cancer and asthma. Similarly, antagonistic drugs reach smaller effects compared to the prediction from their individual potencies. The advantage of combining synergetic drugs is that they can reach higher effects while having lower side effects or toxicity in comparison to a single drug. Its non-parametric and probabilistic nature allows it to model a wide variety of response patterns.Īssessing synergy and antagonism of chemical compounds has applications in medicine, pharmacology and ecotoxicology. The Hand-GP model is a flexible model to capture synergy. We identify cases in which the HandGP model is preferred and cases in which the MuS圜 model is preferred. We also compare our model to the MuS圜 model as an example of a recent method on these five data sets and on two-drug combination screens: Mott et al. We compare the Hand-GP model to standard synergy models and show that our model performs better on these data sets. We evaluate performance of our model on a simulated data set from Greco, two simulated data sets of our own design and two benchmark data sets from Chou and Talalay. Statistical significance of the difference is assessed from the confidence intervals of the Gaussian process fits. From the monotherapeutic response and the Hand principle, we construct a null reference response and synergy is assessed from the difference between this null reference and the Gaussian process fitted response. We introduce a new logarithmic squared exponential kernel for the Gaussian process which captures the logarithmic dependence of response on dose. We propose the Hand-GP model, a non-parametric model based on the combination of the Hand model with Gaussian processes. All of these models, however, fit the dose–response relationship with a parametric model.

A different approach is taken by the MuS圜 model, which directly fits a generalization of the Hill model to the data. Possible choices of null models are Loewe additivity, Bliss independence and the recently rediscovered Hand model. Synergy is usually assessed by comparing the response of drug combinations to a predicted non-interactive response from reference (null) models. Understanding the synergetic and antagonistic effects of combinations of drugs and toxins is vital for many applications, including treatment of multifactorial diseases and ecotoxicological monitoring.
